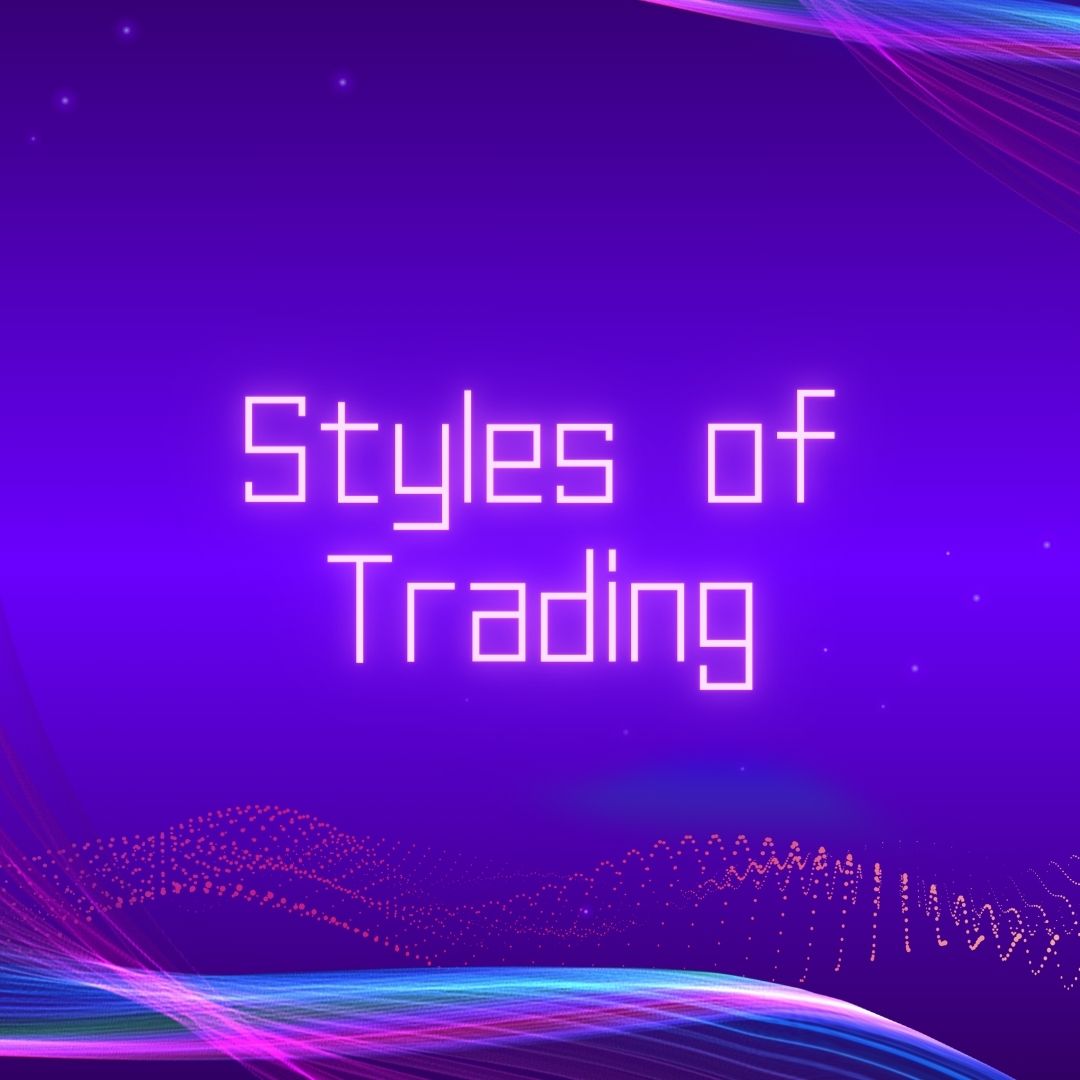
Quantitative Trading: The Basics
Nov 2, 2023 |
Quantitative Trading Strategy
A quantitative trading strategy involves utilizing quantitative models, data analysis techniques, and technical analysis to inform trading decisions. Here are some key elements of a quantitative trading strategy:
1. Data Collection and Analysis: Quantitative traders collect and analyze vast amounts of data from various sources, including economic indicators, market data, company financials, and news. The data is processed and analyzed using statistical models and algorithms to identify patterns and trends.
2. Model Development: Quantitative traders develop mathematical models or algorithms that use historical data to predict future price movements. These models can be based on statistical analysis, machine learning, or other quantitative techniques. The models are continuously refined and optimized using backtesting and other evaluation methods.
3. Risk Management: Strict risk management is essential in quantitative trading. Traders establish risk parameters, such as position sizes and stop-loss levels, to limit potential losses. Risk management techniques are implemented to ensure that trades are executed within defined risk limits.
4. Trading Execution: Quantitative traders utilize automated trading systems to execute trades based on pre-defined rules and algorithms. These systems are typically connected to the market through electronic trading platforms, which allow for rapid execution and access to multiple markets.
5. Monitoring and Optimization: Quantitative traders constantly monitor the performance of their models and strategies. They assess the accuracy and effectiveness of their models, make necessary adjustments, and optimize parameters to adapt to changing market conditions. Regular backtesting and forward testing are conducted to evaluate and refine the trading strategy.
6. High-Frequency Trading (HFT): Quantitative trading often involves high-frequency trading strategies where trades are executed at extremely high speeds. This requires advanced technology infrastructure and low-latency connections to markets.
7. Collaboration with Domain Experts: Quantitative traders often work closely with domain experts, such as economists or industry specialists, to incorporate fundamental data or insights into their models or strategies.
Quantitative trading strategies vary in complexity, ranging from simple rule-based approaches to more sophisticated machine learning algorithms. The success of a quantitative trading strategy relies on the robustness of the models, the quality of the data, and effective risk management practices.
Quantitative Trading Data
The success of quantitative trading relies heavily on the quality and relevance of the data used in the analysis. Here are some key considerations regarding data in quantitative trading:
1. Data Sources: Traders need access to a wide range of data sources, including market data, economic indicators, company financials, news feeds, and social media sentiment. Data can be obtained from various providers, exchanges, financial news platforms, and proprietary sources.
2. Data Quality: Data must be reliable, accurate, and up-to-date to ensure the accuracy of quantitative models and analysis. Traders should ensure that the data they use is sourced from reputable providers and undergoes thorough validation and cleansing processes.
3. Historical Data Analysis: Historical data is crucial for developing and backtesting quantitative models. Traders analyze historical price, volume, and other relevant data to identify patterns, trends, and correlations that can inform trading decisions. This data should cover a sufficiently long time period to capture diverse market conditions and allow for thorough analysis.
4. Real-time and Tick Data: Real-time data is important for executing trades and monitoring positions. Traders require access to real-time price updates, order book data, and other market information to respond quickly to market dynamics. Tick data, which provides detailed information about each individual trade, is valuable for accurate analysis of price movements and short-term trading strategies.
5. News and Event Data: News and event data can have significant impacts on the market. Traders need access to timely news feeds and event calendars to capture market reactions and adjust their trading strategies accordingly. Natural language processing (NLP) techniques may be used to extract relevant information from news articles or social media feeds.
6. Alternative Data: Traders seeking a competitive advantage may utilize alternative data sources, such as satellite imagery, sensor data, web scraping, or credit card transactions, to gain insights into specific industries or companies. Analyzing alternative data can provide unique perspectives and generate alpha in the trading strategy.
Quantitative trading requires continuous access to reliable and up-to-date data from various sources relevant to the markets being traded. Traders should pay attention to data quality, validate and cleanse data, use appropriate historical and real-time data, and consider alternative data sources when applicable. By ensuring the accuracy and relevance of the data, traders can make more informed decisions and generate robust quantitative trading strategies.
Quantitative Trading vs. Other Styles of Trading
Understanding the distinctions between quantitative trading and other styles of trading is crucial for traders. Here are some key differences to consider:
1. Quantitative Trading: Quantitative trading relies heavily on data analysis, mathematical models, and algorithms to make trading decisions. Traders use statistical analysis, machine learning, and computer-based strategies to identify patterns and generate signals. It is a systematic approach driven by quantitative models rather than relying on intuition or gut feel.
2. Discretionary Trading: Discretionary trading involves making trading decisions based on a trader's subjective judgment and interpretation of market conditions. Traders who use discretionary trading rely on their experience, market knowledge, and qualitative factors to make trading decisions. It involves more subjective and non-algorithmic decision-making compared to quantitative trading.
3. Technical Trading: Technical trading focuses on analyzing price charts, patterns, and indicators to make trading decisions. Technical traders rely on historical price data, support and resistance levels, and technical indicators to identify trade entry and exit points. They may incorporate elements of quantitative analysis but may also use subjective judgment in their decision-making process.
4. Fundamental Trading: Fundamental trading involves analyzing company financials, industry trends, and macroeconomic factors to make trading decisions. Fundamental traders assess a company's intrinsic value, growth prospects, and financial health to determine whether a security is overvalued or undervalued. This style of trading relies less on data-driven models and more on qualitative analysis.
It is important for traders to understand these different trading styles and select the approach that aligns with their personal preferences, skills, and risk tolerance. Combining different trading styles or incorporating elements of quantitative analysis within discretionary or technical trading can also be beneficial. Lastly, it is essential to differentiate between trading and investing and to avoid treating investments like trades or trades like investments, as each requires a distinct approach and mindset.
The Bottom Line
In summary, quantitative trading is a data-driven approach to financial trading that relies on mathematical models and algorithms to make trading decisions. While it has traditionally been associated with large financial institutions and hedge funds, technological advancements have made it more accessible to individual traders.
Quantitative trading offers the potential for systematic and disciplined trading strategies based on rigorous data analysis. By utilizing quantitative models, statistical analysis, and algorithms, traders can identify patterns and signals in market data to make informed trading decisions.
However, it is important to note that quantitative trading is not without its risks. The accuracy and effectiveness of models and algorithms can be impacted by changing market conditions or unforeseen events. Proper risk management and ongoing evaluation of the trading strategy are crucial to mitigate risks and adapt to evolving market dynamics.
Ultimately, individual traders should carefully consider their goals, risk tolerance, and expertise before pursuing quantitative trading. Gaining a solid understanding of quantitative techniques, data analysis, and market dynamics is essential for successful implementation.